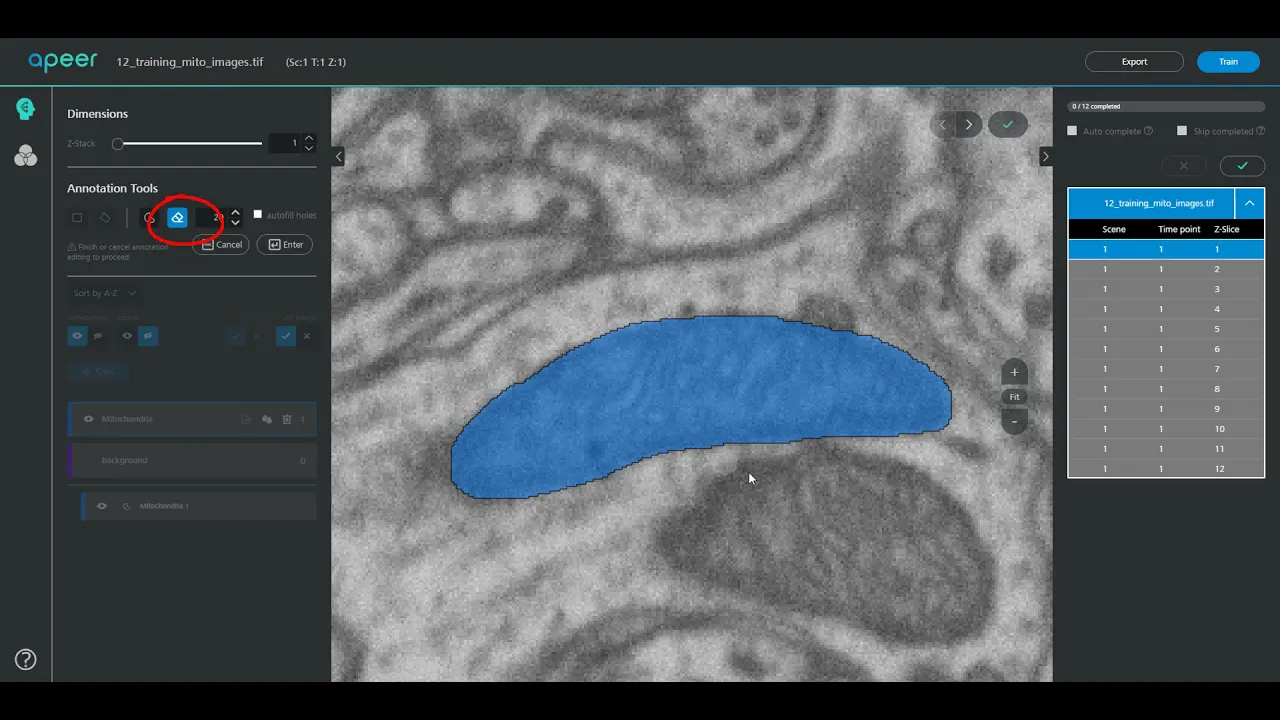
Role
UX/UI designer
Target
B2C
Year
2019
Duration
6 months
Skills
Sketch
Post-it

The target audience for Apeer includes scientists, researchers, and students in the biomedical, pharmaceutical, and life sciences fields who rely on microscopy imaging but often lack coding or machine learning expertise. From cell biologists and pathologists to neuroscience students, these users need intuitive, no-code tools to analyze large image datasets efficiently and accurately. Apeer meets their needs with AI-powered, cloud-based solutions that automate image segmentation and data extraction, enabling faster, more reproducible research and learning without technical barriers.
As a UX designer, I was tasked with introducing a complex machine learning workflow—annotate, train, and segment—to non-technical users. The topic itself was challenging, and I had to design the experience as an MVP using only pre-existing components and patterns from the UI library. My focus was on simplifying the process without losing depth, using clear guidance, visual feedback, and progressive disclosure to make each step intuitive. The goal was to make AI feel less like a black box and more like a powerful, accessible tool.
My Role & Responsibilities
As the sole UX/UI designer, I:
Conducted user interviews, built personas, and synthesized research to frame the user experience.
Translated complex ML concepts into a user-friendly experience
Visualised task analysis and developed user flows to simplify the machine learning process (annotate, train, segment).
Improved the UX in the in-house annotation tool
Designed the MVP experience using pre-existing UI components and patterns, while navigating strict limitations from business and development teams.
Collaborated with product managers and developers to ensure technical feasibility and maintain a balance between user needs and technical constraints.
Advocated for non-technical users by simplifying language, interactions, and visual feedback.
To deeply understand the user experience around machine learning in microscopy, I conducted interviews with 6 participants across different levels of technical expertise. After each session, I mapped out the insights on a shared board using post-its, organizing observations around key moments in the workflow. This visual mapping allowed patterns and recurring pain points to emerge quickly.
Through this process, I identified a clear sequence of core steps users needed to go through: annotating datasets, training the model, receiving augmented data, processing 2D segmentations, and finally extracting insights. Each of these steps came with its own set of mental models, expectations, and areas of uncertainty.
To make sense of the complexity, I created a synthesis of the interviews that distilled user goals, frustrations, and informational gaps. This synthesis helped me uncover what users needed to know at each step and where they struggled most—especially with confidence, understanding outcomes, and knowing what would happen next.
I then built a detailed task analysis of the entire machine learning process, breaking it down step by step from the user's point of view. This task analysis became a foundational reference that guided design decisions throughout the project. It helped me align the interface with users’ mental models and ensure that each part of the experience felt purposeful, clear, and supportive—even within the tight constraints of our MVP.
As a UX designer, I synthesized all the interviews to create an ideal workflow representation, which served as a blueprint for the entire experience. I broke down the workflow into distinct tasks to ensure clarity and efficiency at each step. This task analysis distills the ML workflow into three actionable jobs—Annotate, Train, and Process (for Segmentation). For each phase, I identified core tasks, cognitive load, and pain points, then streamlined micro‑interactions to reduce repetition and decision fatigue. The result: non‑technical users can label a small image set, fine-tune parameters with guided defaults, and batch-process thousands of images—all while feeling confident and in control

The following videos were created by the APEER costumer support team for e-learning purposes but they are also valuable to explain how I designed the MVP experience. My goal was to reduce cognitive load and make high-quality data labeling accessible without requiring ML expertise.
Annotate
In the initial phase of the machine learning workflow, I focused on creating a user-friendly annotation interface that simplifies the process of labeling images for AI training. Understanding that accurate annotations are critical for model performance, I designed tools that allow users to effortlessly draw bounding boxes and apply labels. To enhance efficiency, I incorporated features such as keyboard shortcuts and zoom functionalities, enabling users to navigate and annotate large datasets with ease. The interface was tested with end-users to ensure that it met their needs and allowed for quick, precise annotations, laying a solid foundation for the subsequent training phase.
Apeer tutorial for annotate phase
Train
Transitioning to the training phase, I aimed to demystify the model training process for users by providing clear, actionable feedback. The interface displays real-time updates on training progress, including metrics like accuracy and loss, presented through intuitive visualizations. Users can easily adjust parameters and initiate retraining, fostering an interactive environment that encourages experimentation and learning. By simplifying complex processes into comprehensible steps, the design empowers users to engage confidently with model training, regardless of their technical background.
Sneak peek of training phase
Apeer tutorial for training phase
Segment
In the final segmentation phase, the focus was on enabling users to apply trained models to new data effectively. The interface allows users to upload images and view segmentation results, with options to fine-tune outputs as needed. I integrated features that let users compare original images with segmented outputs side by side, facilitating a deeper understanding of model performance. This design ensures that users can validate and refine segmentation results, closing the loop in the machine learning workflow and promoting continuous improvement.
Apeer tutorial for segmentation phase